Guiding Principles for Transparent Machine Learning in Medical Devices
In 2021, the FDA, Health Canada, and the UK’s MHRA jointly identified 10 guiding principles for good machine learning practice (GMLP) to ensure the development of safe, effective, and high-quality AI/ML technologies in healthcare. These principles promote continuous learning from real-world use and performance improvements in medical devices.
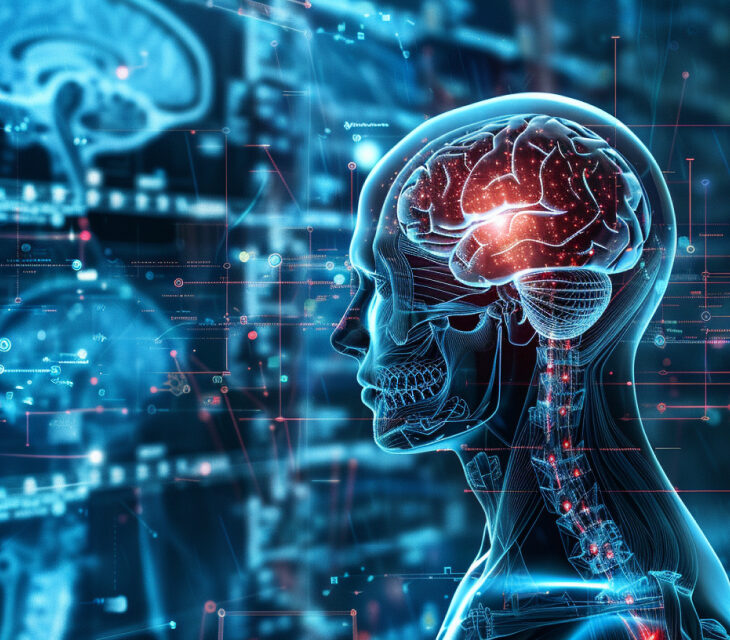
Key Transparency Principles for Machine Learning Enabled Medical Devices
Building on the GMLP principles, particularly focusing on the human-AI team performance and providing users with clear, essential information, these transparency principles aim to enhance safety, effectiveness, and trust in MLMDs.
What is Transparency?
Transparency involves clearly communicating the device’s intended use, development process, performance, and decision logic to relevant audiences. This includes:
- How an output or decision was reached.
- Making the decision logic understandable.
- Effective Transparency
- Communicate information impacting risks and patient outcomes.
- Provide relevant information to the intended audience considering the context.
- Use appropriate media, timing, and strategies for successful communication.
- Employ a holistic understanding of users, environments, and workflows.
Human-Centered Design
A crucial aspect of transparency is human-centered design, an iterative process involving all relevant parties throughout the design and development phases. This approach ensures that MLMDs are transparent, validated, and provide users with all necessary information.
Transparency Guiding Principles
Who: Transparency should be provided to healthcare professionals, patients, caregivers, support staff, administrators, payers, and governing bodies.
Why: Transparency is vital for patient-centered care, safety, and effective device usage. It helps identify and manage risks, supports informed decision-making, and promotes trust and confidence in MLMDs.
What: Share information about the device’s medical purpose, function, intended users, use environments, target populations, performance, benefits, risks, and development processes. Include details on the device’s logic, biases, limitations, and risk management strategies.
Where: Optimize the software user interface to be responsive, personalized, and adaptive, addressing user needs through various modalities like audio, video, on-screen text, alerts, diagrams, and document libraries.
When: Provide detailed information throughout the product lifecycle, timely updates on modifications, and notifications about new information. Offer targeted instructions or warnings during specific workflow stages or triggers.
How: Apply human-centered design principles to ensure information is accessible and usable. Use appropriate detail levels and content arrangement to support user understanding and decision-making.
These guiding principles for transparency in machine learning medical devices are intended to support the adoption and advancement of good transparency practices. Continuous engagement and collaboration in this field will help develop and implement effective transparency standards.
Find out more: